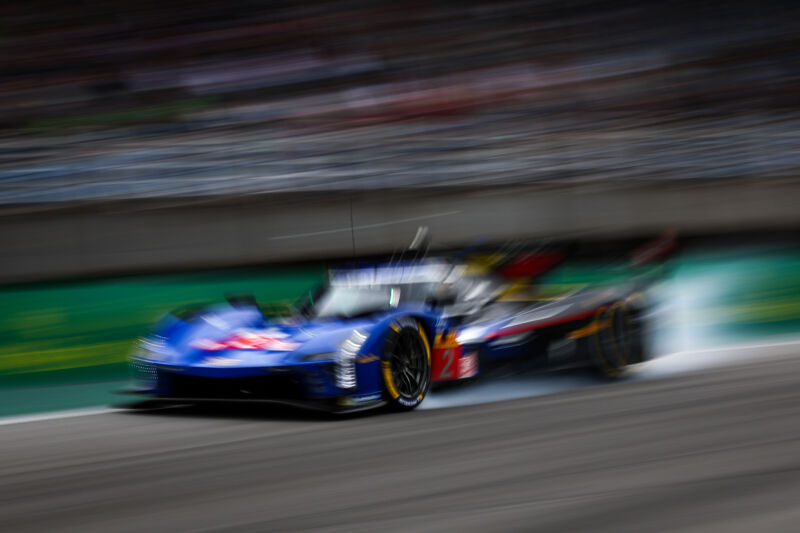
James Moy Photography/Getty Images
It is hard to escape the feeling that a few too many businesses are jumping on the AI hype train because it’s hype-y, rather than because AI offers an underlying benefit to their operation. So I will admit to a little inherent skepticism, and perhaps a touch of morbid curiosity, when General Motors got in touch wanting to show off some of the new AI/ML tools it has been using to win more races in NASCAR, sportscar racing, and IndyCar. As it turns out, that skepticism was misplaced.
GM has fingers in a lot of motorsport pies, but there are four top-level programs it really, really cares about. Number one for an American automaker is NASCAR—still the king of motorsport here—where Chevrolet supplies engines to six Cup teams. IndyCar, which could once boast of being America’s favorite racing, is home to another six Chevy-powered teams. And then there’s sportscar racing; right now Cadillac is competing in IMSA’s GTP class and the World Endurance Championship’s Hypercar class, plus a factory Corvette Racing effort in IMSA.
“In all the series we race we either have key partners or specific teams that run our cars. And part of the technical support that they get from us are the capabilities of my team,” said Jonathan Bolenbaugh, motorsports analytics leader at GM, based at GM’s Charlotte Technical Center in North Carolina.
Unlike generative AI that’s being developed to displace humans from creative activities, GM sees the role of AI and ML as supporting human subject-matter experts so they can make the cars go faster. And it’s using these tools in a variety of applications.
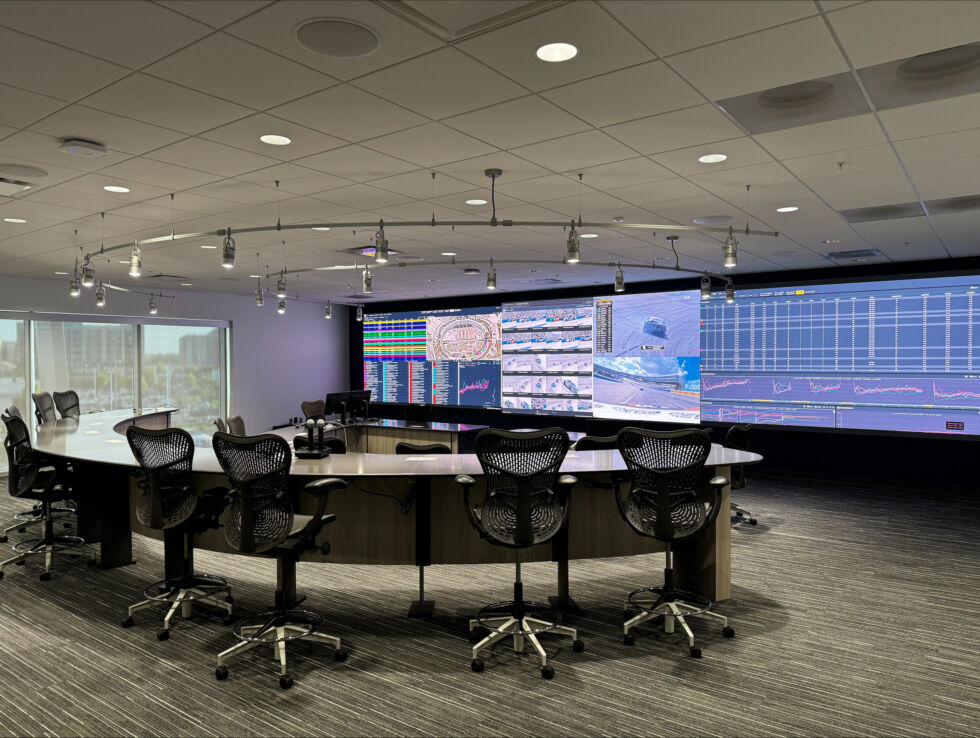
General Motors
Each team in each of those various series (obviously) has people on the ground at each race, and invariably more engineers and strategists helping them from Indianapolis, Charlotte, or wherever it is that the particular race team has its home base. But they’ll also be tied in with a team from GM Motorsport, working from one of a number of command centers at its Charlotte Technical Center.
What did they say?
Connecting all three are streams and streams of data, from the cars themselves (in series that allow car-to-pit telemetry) but also voice comms, text-based messaging, timing and scoring data from officials, trackside photographs, and more. And one thing Bolenbaugh’s team and their suite of tools can do is help make sense of that data quickly enough for it to be actionable.
“In a series like f1, a lot of teams will have students who are potentially newer members of the team literally listening to the radio and typing out what is happening, then saying, ‘hey, this is about pitting. This is about track conditions,'” Bolenbaugh said.
Instead of giving that to the internship kids, GM built a real time audio transcription tool to do that job. After trying out a commercial off-the-shelf solution, it decided to build its own, “a combination of open source and some of our proprietary code,” Bolenbaugh said. As anyone who has ever been to a race track can attest, it’s a loud environment, so GM had to train models with all the background noise present.
“We’ve been able to really improve our accuracy and usability of the tool to the point where some of the manual support for that capability is now dwindling,” he said, with the benefit that it frees up the humans, who would otherwise be transcribing, to apply their brains in more useful ways.
Take a look at this
Another tool developed by Bolenbaugh and his team was built to quickly analyze images taken by trackside photographers working for the teams and OEMs. While some of the footage they shoot might be for marketing or PR, a lot of it is for the engineers.
Two years ago, getting those photos from the photographer’s camera to the team was the work of two to three minutes. Now, “from shutter click at the racetrack in a NASCAR event to AI-tagged into an application for us to get information out of those photos is seven seconds,” Bolenbaugh said.
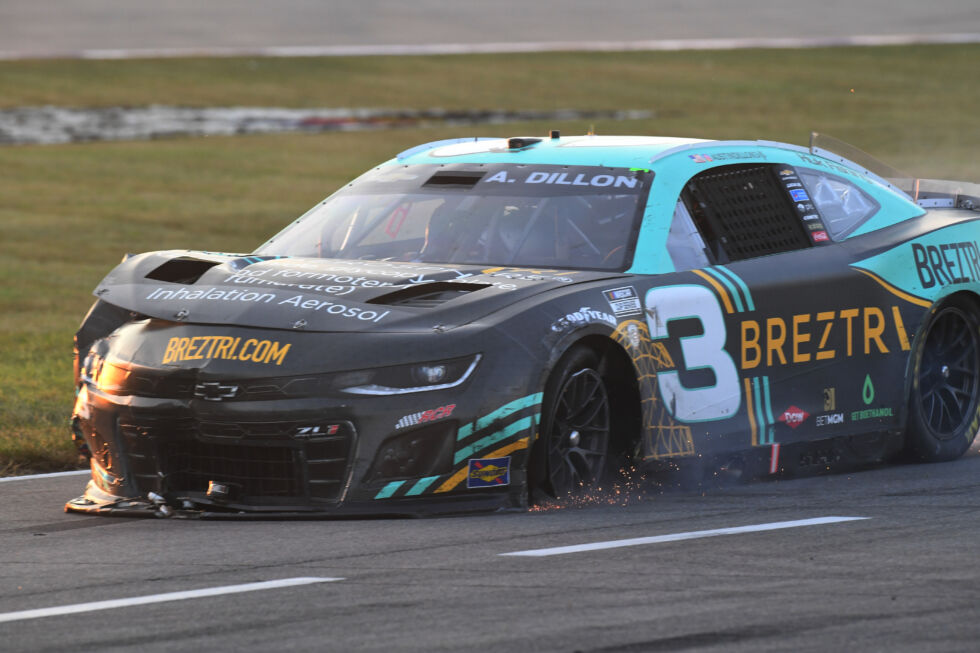
Jeffrey Vest/Icon Sportswire via Getty Images
“Time is everything, and the shortest lap time that we run—the Coliseum would be an outlier, but maybe like 18 seconds is probably a short lap time. So we need to be faster than from when they pass that pit lane entry to when they come back again,” he said.
At the rollout of this particular tool at a NASCAR race last year, one of GM’s partner teams was able to avoid a cautionary pitstop after its driver scraped the wall, when the young engineer who developed the tool was able to show them a seconds-old photo of the right side of the car that showed it had escaped any damage.
“They didn’t have to wait for a spotter to look, they didn’t have to wait for the driver’s opinion. They knew that didn’t have damage. That team made the playoffs in that series by four points, so in the event that they would have pitted, there’s a likelihood where they didn’t make it,” he said. In cases where a car is damaged, the image analysis tool can automatically flag that and make that known quickly through an alert.
Not all of the images are used for snap decisions like that—engineers can glean a lot about their rivals from photos, too.
“We would be very interested in things related to the geometry of the car for the setup settings—wicker settings, wing angles… ride heights of the car, how close the car is to the ground—those are all things that would be great to know from an engineering standpoint, and those would be objectives that we would have in doing image analysis,” said Patrick Canupp, director of motorsports competition engineering at GM.
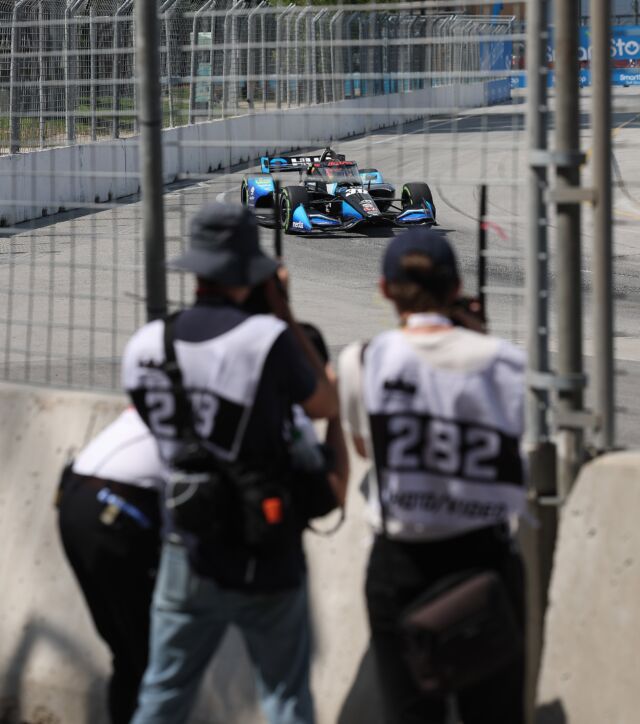
Steve Russell/Toronto Star via Getty Images
“It’s not straightforward to take a set of still images and determine a lot of engineering information from those. And so we’re working on that actively to help with all the photos that come in to us on a race weekend—there’s thousands of them. And so it’s a lot of information that we have at our access, that we want to try to maximize the engineering information that we glean from all of that data. It’s kind of a big data problem that AI is really geared for,” Canupp said.
The computer says we should pit now
Remember that transcribed audio feed from earlier? “If a bunch of drivers are starting to talk about something similar in the race like the track condition, we can start inferring, based on… the occurrence of certain words, that the track is changing,” said Bolenbaugh. “It might not just be your car… if drivers are talking about something on track, the likelihood of a caution, which is a part of our strategy model, might be going up.”
That feeds into a strategy tool that also takes lap times from timing and scoring, as well as fuel efficiency data in racing series that provide it for all cars, or a predictive model to do the same in series like NASCAR and IndyCar where teams don’t get to see that kind of data from their competitors, as well as models of tire wear.
“One of the biggest things that we need to manage is tires, fuel, and laptime. Everything is a trade off between trying to execute the race the fastest,” Bolenbaugh said.
Obviously races are dynamic situations, and so “multiple times a lap as the scenario changes, we’re updating our recommendation. So, with tire fall off [as the tire wears and loses grip], you’re following up in real-time, predicting where it’s going to be. We are constantly evolving during the race and doing transfer learning so we go into the weekend, as the race unfolds, continuing to train models in real time,” Bolenbaugh said.